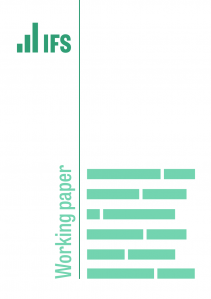
Advancing microdata models and methods
Showing 97 - 108 of 210 results
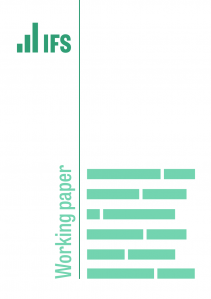
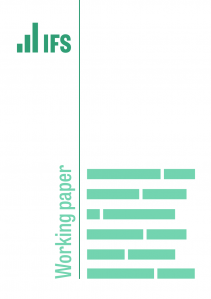
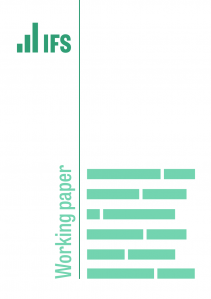
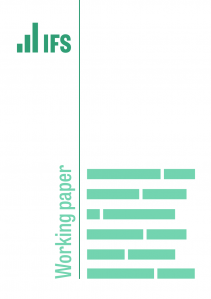
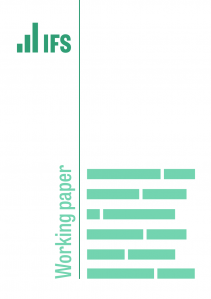
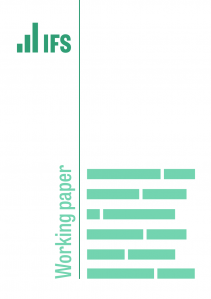
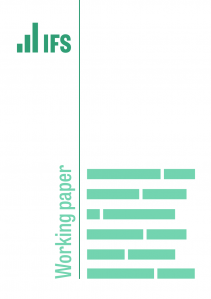
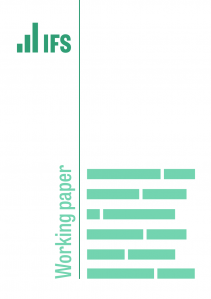
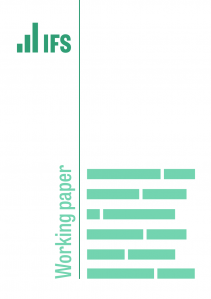
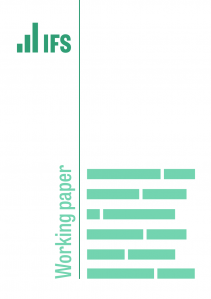
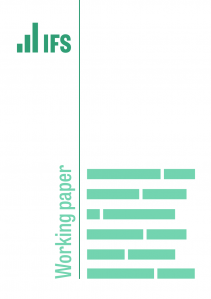
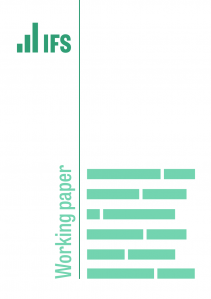
This paper derives conditions under which preferences and technology are nonparametrically identified in hedonic equilibrium models, where products are differentiated along more than one dimension and agents are characterized by several dimensions of unobserved heterogeneity.
11 June 2019
We propose a bootstrap-based calibrated projection procedure to build confidence intervals for single components and for smooth functions of a partially identified parameter vector in moment (in)equality models. The method controls asymptotic coverage uniformly over a large class of data generating processes.
7 June 2019
Econometrics has traditionally revolved around point identication. Much effort has been devoted to finding the weakest set of assumptions that, together with the available data, deliver point identification of population parameters, finite or infinite dimensional that these might be. And point identification has been viewed as a necessary prerequisite for meaningful statistical inference. The research program on partial identification has begun to slowly shift this focus in the early 1990s, gaining momentum over time and developing into a widely researched area of econometrics.
31 May 2019
To perform Bayesian analysis of a partially identified structural model, two distinct approaches exist: standard Bayesian inference, which assumes a single prior for the structural parameters, including the non-identified ones; and multiple-prior Bayesian inference, which assumes full ambiguity for the non-identified parameters. The prior inputs considered by these two extreme approaches can often be a poor representation of the researcher’s prior knowledge in practice.
28 May 2019
This paper describes a method for carrying out non-asymptotic inference on partially identified parameters that are solutions to a class of optimization problems. The optimization problems arise in applications in which grouped data are used for estimation of a model's structural parameters. The parameters are characterized by restrictions that involve the population means of observed random variables in addition to the structural parameters of interest. Inference consists of finding confidence intervals for the structural parameters. Our method is non-asymptotic in the sense that it provides a finite-sample bound on the difference between the true and nominal probabilities with which a confidence interval contains the true but unknown value of a parameter. We contrast our method with an alternative non-asymptotic method based on the median-of-means estimator of Minsker (2015). The results of Monte Carlo experiments and an empirical example illustrate the usefulness of our method.
17 May 2019
There exists a useful framework for jointly implementing Durbin-Wu-Hausman exogeneity and Sargan-Hansen overidentication tests, as a single articial regression. This note sets out the framework for linear models and discusses its extension to non-linear models. It also provides an empirical example and some Monte Carlo results.
16 May 2019
Factor structures or interactive effects are convenient devices to incorporate latent variables in panel data models. We consider fixed effect estimation of nonlinear panel single-index models with factor structures in the unobservables, which include logit, probit, ordered probit and Poisson specications.
11 April 2019
This paper examines the asymptotic behavior of the posterior distribution of a possibly nondifferentiable function g(θ), where θ is a finite-dimensional parameter of either a parametric or semiparametric model. The main assumption is that the distribution of a suitable estimator θ^n, its bootstrap approximation, and the Bayesian posterior for θ all agree asymptotically.
3 April 2019
This paper considers inference on fixed effects in a linear regression model estimated from network data.
3 April 2019
We propose to combine smoothing, simulations and sieve approximations to solve for either the integrated or expected value function in a general class of dynamic discrete choice (DDC) models.
3 April 2019
In this paper we investigate panel regression models with interactive fixed effects. We propose two new estimation methods that are based on minimizing convex objective functions.
3 April 2019
Efron's elegant approach to g-modeling for empirical Bayes problems is contrasted with an implementation of the Kiefer-Wolfowitz nonparametric maximum likelihood estimator for mixture models for several examples. The latter approach has the advantage that it is free of tuning parameters and consequently provides a relatively simple complementary method.
3 April 2019