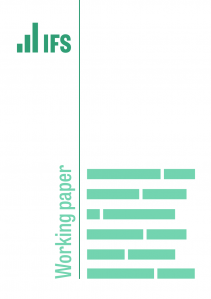
Advancing microdata models and methods
Showing 85 - 96 of 210 results
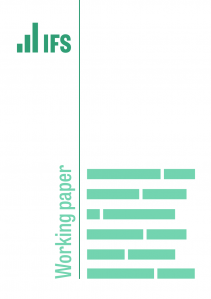
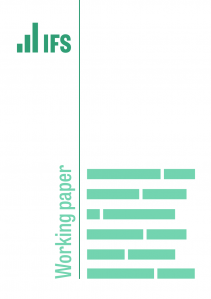
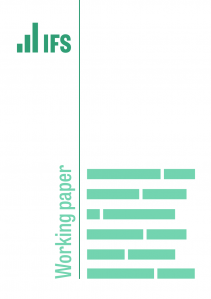
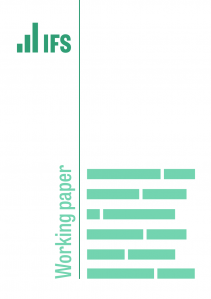
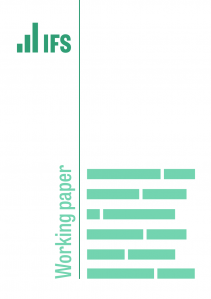
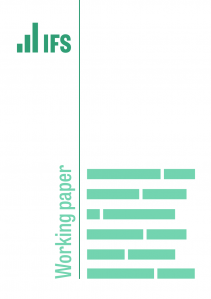
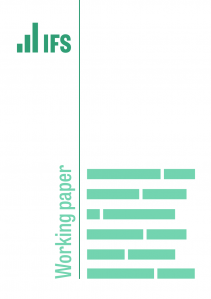
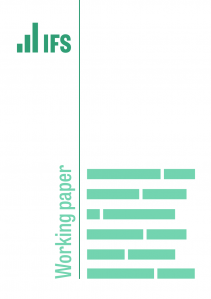
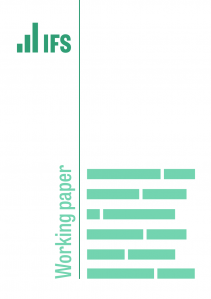
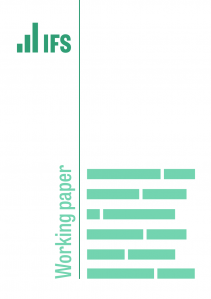
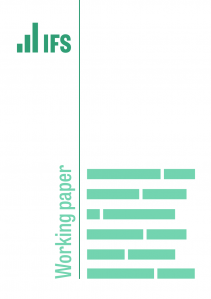
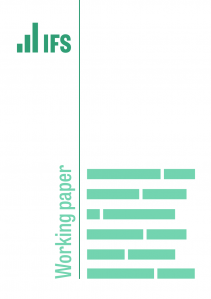
We study nonparametric estimation of density functions for undirected dyadic random variables (i.e., random variables defined for all n def = (N/2) unordered pairs of agents/nodes in a weighted network of order N).
7 August 2019
We develop methods for robust Bayesian inference in structural vector autoregressions (SVARs) where the impulse responses or forecast error variance decompositions of interest are set-identified using external instruments (or ‘proxy SVARs’).
23 July 2019
We propose a robust method of discrete choice analysis when agents’ choice sets are unobserved. Our core model assumes nothing about agents’ choice sets apart from their minimum size.
5 July 2019
This paper introduces measures for how each moment contributes to the precision of the parameter estimates in GMM settings.
5 July 2019
This paper develops identification and estimation methods for dynamic structural models when agents’ actions are unobserved by econometricians. We provide conditions under which choice probabilities and latent state transition rules are nonparametrically identified with a continuous state variable in a single-agent dynamic discrete choice model.
18 June 2019
We consider off-policy evaluation and optimization with continuous action spaces. We focus on observational data where the data collection policy is unknown and needs to be estimated. We take a semi-parametric approach where the value function takes a known parametric form in the treatment, but we are agnostic on how it depends on the observed contexts.
12 June 2019
The relationship between democracy and economic growth is of long standing interest. We revisit the panel data analysis of this relationship by Acemoglu et al. (forthcoming) using state of the art econometric methods. We argue that this and lots of other panel data settings in economics are in fact high-dimensional, resulting in principal estimators – the fixed effects (FE) and Arellano-Bond (AB) estimators – to be biased to the degree that invalidates statistical inference.
12 June 2019
This paper studies inference on treatment effects in aggregate panel data settings with a single treated unit and many control units. We propose new methods for making inference on average treatment effects in settings where both the number of pre-treatment and the number of post-treatment periods are large.
12 June 2019
We study a panel data model with general heterogeneous effects, where slopes are allowed to be varying across both individuals and times. The key assumption for dimension reduction is that the heterogeneous slopes can be expressed as a factor structure so that the high-dimensional slope matrix is of low-rank, so can be estimated using low-rank regularized regression.
12 June 2019
Due to the increasing availability of high-dimensional empirical applications in many research disciplines, valid simultaneous inference becomes more and more important. For instance, high-dimensional settings might arise in economic studies due to very rich data sets with many potential covariates or in the analysis of treatment heterogeneities.
12 June 2019
Graphical models have become a very popular tool for representing dependencies within a large set of variables and are key for representing causal structures. We provide results for uniform inference on high-dimensional graphical models with the number of target parameters d being possible much larger than sample size.
12 June 2019
This paper considers inference for a function of a parameter vector in a partially identified model with many moment inequalities. This framework allows the number of moment conditions to grow with the sample size, possibly at exponential rates. Our main motivating application is subvector inference, i.e., inference on a single component of the partially identified parameter vector associated with a treatment effect or a policy variable of interest.
12 June 2019